Kundenzentrierung mit Sentiment Analysis
Ein zufriedener Kunde ist die Basis für Geschäftserfolg. Dazu müssen wir verstehen, was der Kunde erreichen will und was ihn daran hindert.
Autor: Amancio Bouza
Das Kundenproblem muss identifiziert und eine Lösung angeboten werden.
Das ist jedoch leichter gesagt, als getan. Ein Beispiel aus der Tourismus-Branche: Anita bucht bei einer Reise-Agentur eine Flussfahrt auf der Seine bis nach Paris für zwei Personen. Sie geht auf diese Reise und kehrt anschliessend wieder in die Agentur zurück, um dort ihr Feedback abzugeben.
Mit welcher Ja/Nein Frage kann die Agentur herausfinden, was Anita’s Ziele dabei waren, ob sie diese erreicht hat und wenn nicht, warum nicht? Dazu müssten geschlossene Fragen in dieser Art gestellt werden:
- Wollten Sie auf den Eiffelturm hoch?
- Hat es geklappt?
- Wenn nein, war die Warteschlange zu lang?
- Sind Sie mit dem Eiffelturm zufrieden?
Geschlossene Fragen bzw. Ja/Nein Antworten können schnell quantitativ ausgewertet werden. Aber wurden die richtigen Fragen gestellt? Konnte der Kunde mit Ja/Nein seine Meinung und emotionale Haltung ausdrücken?
Einfacher geht es, wenn nur folgende zwei (offene) Fragen gestellt werden:
- Was wollten Sie mit der Reise erreichen?
- Wenn Sie es nicht erreicht haben, warum nicht?
Von Anita bekommt die Agentur eine natürlichsprachliche Antwort. Solche Antworten enthalten Äusserungen, was das Ziel ihrer Reise war, ob sie es erreicht hat und wenn nicht, was sie daran gehindert hat. Solche Antworten sind aufwendig auszuwerten, da man sie nicht einfach aufsummieren kann. Im Gegensatz zu vorhin liefern sie aber ein qualitativ hochwertiges Feedback.
An dieser Stelle kommt Sentiment Analysis ins Spiel. Es ist ein Verfahren, welches die Auswertung von natürlichsprachlichen Aussagen automatisiert analysiert, Kernaussagen oder Meinungen identifiziert und diese bzgl. emotionaler Haltung (engl.: sentiment) klassifiziert.
Im Folgenden werden drei Geschäftsnutzen aufgezeigt, welche durch Sentiment Analysis erreicht werden können: eine höhere Kundenzufriedenheit durch verbesserte Produkte, das Erkennen von Problemen in Real-Time und eine Differenzierung im Markt. Danach wird aufgezeigt, was Sentiment Analysis ist und wie es grundsätzlich funktioniert. Abschliessend folgt eine Zusammenfassung.
Geschäftsnutzen von Sentiment Analysis
Neue Technologien wie Artificial Intelligence (AI) und Sentiment Analysis sind technisch interessant. Wenn wir daraus Geschäftsnutzen erzeugen können, dann wird’s spannend! Drei solche Geschäftsnutzen werden hier umrissen.
Höhere Kundenzufriedenheit durch verbesserte Produkte
Mit natürlichsprachlichen Kommentaren beschreibt der Kunde seine Erfahrung mit einem Produkt und bringt seine Meinung und Haltung dazu zum Ausdruck. Mittels Sentiment Analysis können wir diese Kommentare genau untersuchen. Dabei identifizieren wir Problemfelder, eruieren Kernaussagen und Meinungen und klassifizieren diese bzgl. der emotionalen Haltung. So erhalten wir wertvolle Insights, ob der Kunde zufrieden ist und wie wir das Produkt ggf. verbessern sollten.
Zum Beispiel: Anita sagt: «Wir wollten unser romantisches Wochenende nicht in der Warteschlange beim Eiffelturm verbringen.» Es wird eine negative Haltung bzgl. der Warteschlange beim Eiffelturm identifiziert. Die Agentur könnte nun das Reiseangebot mit einem Skip-The-Line Ticket erweitern und damit verbessern.
In Real-Time Probleme erkennen und agieren
Heutzutage kann ein Kunde seine Unzufriedenheit schnell über Social Media der ganzen Welt mitteilen. Daraus ergeben sich zwei Problemfelder:
- Der Kunde ist verärgert und wählt einen neuen Anbieter.
- Sein öffentlicher Kommentar schadet der Marke und Reputation und erschwert dem Anbieter damit, Neukunden zu gewinnen.
Diese Form von Feedback ist aber auch eine Chance! Denn wir können mittels Sentiment Analysis auch auf Social Media automatisiert Kommentare in Echtzeit interpretieren und Massnahmen einleiten, um die Kundenzufriedenheit zu erhöhen.
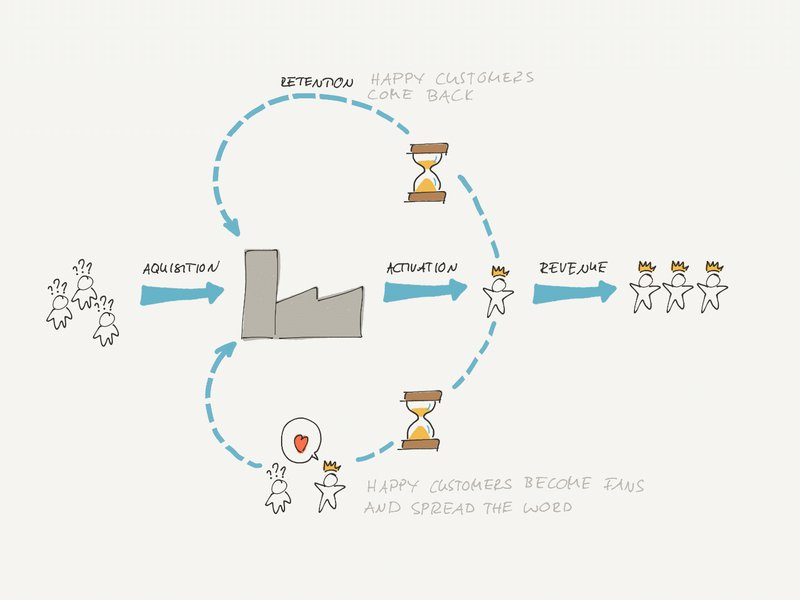
Zum Beispiel: Anita ist gerade in Paris und sie beklagt sich auf Facebook: «Paris habe ich mir romantischer vorgestellt.». Die Agentur könnte diese Information an die Hotel-Rezeption weiterleiten mit dem Verweis, Anita am folgenden Tag einen Geheim-Tipp für einen romantischen Ausflug zu geben: «Geniessen Sie ein Picknick im Jardin du Luxembourg.». Anita wäre nicht mehr von Paris enttäuscht oder über die Reise verärgert (1) und würde im Idealfall auch noch einen romantischen, glücklichen, zufriedenen Post/Kommentar über ihr Picknick veröffentlichen (2).
Differenzierung im Markt: Konkurrenz besser verstehen
Öffentliche Kommentare auf Review Portalen beziehen sich oft auch auf Produkte/Angebote der Konkurrenz. Mit Sentiment Analysis kann man die Kunden-Kommentare der Konkurrenz analysieren und zur eigenen Profilierung und Marktpositionierung anwenden.
Wenn wir wissen, was Kunden bei der Konkurrenz besonders schätzen und was nicht, können wir diese Insights nutzen, um uns besser zu differenzieren oder unser Angebot auszuweiten. Kombiniert mit der Analyse von Kundensegmenten, können wir damit bspw. gezielter Marketing-Nachrichten ausliefern.
«Our best competitors reveal our weaknesses. The goal is not to „beat“ our competition but rather to improve ourselves.» Simon Sinek
Zum Beispiel: Anita ist von ihrer Parisreise zurück und schreibt als Review: «Paris war schön. Die Reiseagentur hat mir sehr viele Tipps für Must-See Sehenswürdigkeiten gegeben. Leider habe ich einige verpasst, da mir nicht klar war, dass sie z.T. direkt beieinander lagen.»
Gleichzeitig hat ein Kunde, der über eine andere Reiseagentur gebucht hat, auch ein Review verfasst: «Paris war schön. Die Agentur hat mir eine perfekte Route vorgeschlagen, um möglichst viele nahe beieinander liegende Must-See Sehenswürdigkeiten in kürzester Zeit zu besuchen. Wunderbar!»
Der andere Kunde hat in der gleichen Zeit viel mehr Sehenswürdigkeiten gesehen, als Anita. Der ausschlaggebende Unterschied war, dass er eine Route vorgeschlagen bekam, um alle Sehenswürdigkeiten, die beieinander liegen, zu besichtigen. Anita hat lediglich eine einfache Liste von Sehenswürdigkeiten erhalten. Diese liegen z.T. sehr weit voneinander entfernt bzw. beinhaltete die Liste nicht, welche Sehenswürdigkeiten beieinander sind. Anita’s Agentur kann von der anderen Agentur lernen und auch solche Sehenswürdigkeits-Routen vorschlagen, um es ihren Kunden in Zukunft einfacher zu machen, mehr von Paris zu sehen.
Was ist Sentiment Analysis?
Eine Person hat eine Erfahrung mit einem Produkt gemacht und drückt diese in schriftlicher Form bspw. Als Kommentar aus. Dieser Text beinhaltet: Wer sagt was über wen/was. Mit Sentiment Analysis wird daraus (konkret aus dem «was») eine Meinung (engl. Opinion) extrahiert und die jeweilige emotionale Haltung (engl. Sentiment) dazu klassifiziert.
Um es mit dem Beispiel von Anitas Reise zu erklären:
Anita ist mit ihrem Freund von ihrer Seine-Flussfahrt nach Paris zurückgekehrt. Die Reise war ein Pauschalangebot der Reisefirma Travelito. Anita hat positive und negative Erfahrungen gemacht, die sie nun auf TripAdvisor teilt.
Sie schreibt als Kommentar:

Anita’s Kommentar enthält interessante Informationen. Dazu gehören Themenfelder, Meinungen, Emotionen und Haltungen.
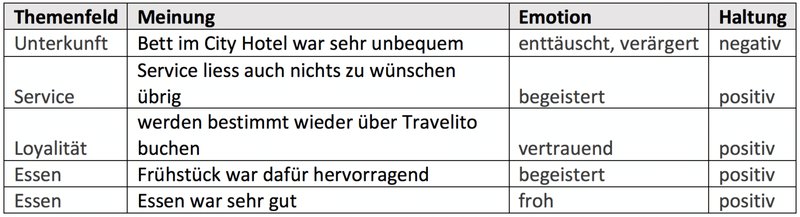
Sentiment Analysis macht es möglich Themenfelder wie Unterkunft, Essen, Service, etc. zu identifizieren, Anita’s Meinungen darüber zu extrahieren, ihre Emotion dazu festzulegen und entsprechend ihre Haltung (Sentiment) zu erkennen, bzw. festzustellen, ob sie damit zufrieden oder unzufrieden ist. Somit erhalten wir ein qualitatives Feedback eines Kunden und können ggf. unser Angebot verbessern.
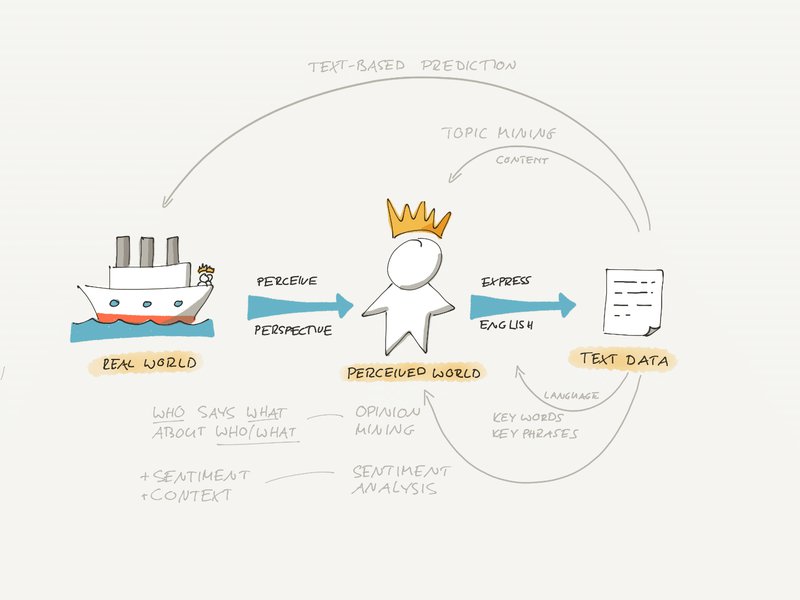
Hätte Anita nur eine Sternchen-Bewertung für einen Net Promoter Score (NPS) angeben können, hätte sie wahrscheinlich eine 7 von 10 angegeben. Dann müssten wir raten, wieso es keine 10 geworden ist bzw. wüssten es einfach nicht. NPS ist somit ein guter KPI (rechnerisch), aber ein schlechter Ratgeber zu Kundenbedürfnis und Anbegotsverbesserungen.
Wie funktioniert Sentiment Analysis?
Im Folgenden soll gezeigt werden, wie Sentiment Analysis eine Meinung aus einem Text extrahiert und ggf. eine Haltung daraus klassifiziert. Zuerst müssen bestimmte Themenfelder organisiert werden. Dann kann die Meinung extrahiert und die Haltung einer Person klassifiziert werden. Durch dieses Sammeln und Analysieren können wertvolle Insights aufgedeckt werden. Diese ermöglichen es, ein Produkt zu verbessern, auf Kundenbedürfnisse zu reagieren und sich effektiver auf dem Markt zu positionieren. Einfachheitshalber werden wesentliche Schritte wie Datenbereinigung und -aufbereitung ausgelassen.
Bestimme Themenfelder
Um grosse Textmengen, insbesondere Kommentare, zu analysieren und zu verstehen, müssen wir diese zuerst entsprechend organisieren. Dazu bestimmen wir als erstes Themenfelder. Dieses Verfahren ist bekannt als Topic Mining.
Themenfelder können explizit genannt sein (z.B. City Hotel = Unterkunft) oder implizit beschrieben werden (z.B. Frühstück = Essen). Je nach Anwendungsfall kann es durchaus Sinn machen, Themenfelder wie City Hotel und Essen zu „Kost und Logis“ zusammenzufassen. Der im vorangehenden Beispiel genannte Kommentar von Anita («Das Bett im City Hotel war sehr unbequem. Das Frühstück war dafür hervorragend, das Essen war sehr gut und der Service liess auch nichts zu wünschen übrig. Wir hatten eine wunderschöne Zeit und werden bestimmt wieder über Travelito buchen.») beinhaltet aber auch ein latentes Themenfeld wie „Kundenloyalität“.
Die Art und Weise, wie man Themenfelder definiert, nennt man Topic Modelling. Die einfachste Art, ein Thema zu definieren, ist über sogenannte Schlüsselwörter (bspw. Hotel, Essen). Es gibt aber auch kompliziertere Verfahren wie Latent Semantic Analysis (LSA) oder Latent Dirichlet Allocation (LDA), um versteckte Themenfelder (bspw. Kundenloyalität) zu identifizieren. Diese werden oftmals durch eine Menge von Wörtern implizit beschrieben, sogenannte Bag of Words. LSA bzw. LDA sind statistische Verfahren, um relevante Bag of Words zu identifizieren.
Nun klassifizieren wir Kommentare und teilen diese einem oder mehreren Themenfeldern zu. Dazu verwenden wir traditionelle Document Retrieval Verfahren.
Das folgende Bild zeigt Themenfelder, welche wir im Auftrag eines internationalen Reiseunternehmens aus echten Kommentaren extrahiert haben. Die Schriftgrösse des Wortes zeigt, wie oft das Wort als Thema in den Kommentaren erwähnt wurde. In diesem Fall haben wir nur negative Kommentare verwertet. Hier sehen wir bspw. schnell, dass es viele negative Kommentare zu Paris, Normandy und Lyon gibt.
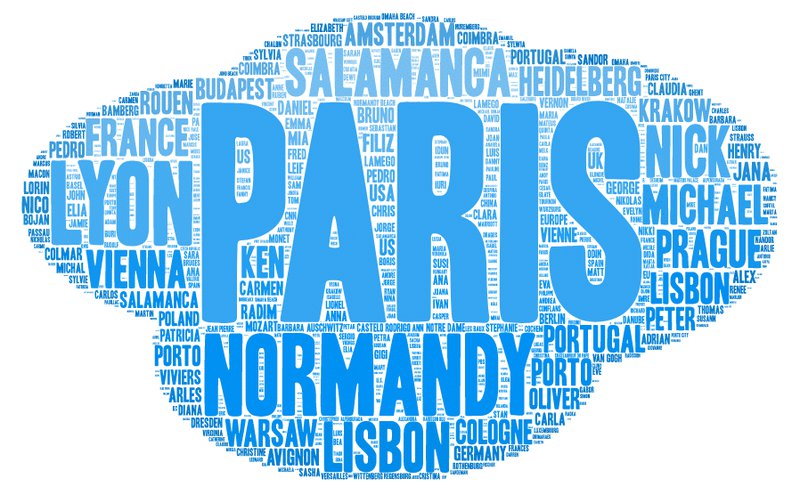
Extrahiere Meinungen
Um aus einem Text Meinungen zu extrahieren, müssen wir in der Lage sein, Sätze wie «Das Bett im City Hotel war sehr unbequem» automatisiert zu analysieren. Das heisst, wir müssen Sätze wie folgt zerlegen können.

Dazu verwenden wir Techniken aus Natural Language Processing und Understanding, wie Part-of-Speech Tagging (bspw. Objekt, Verb, Adjektiv), oder Entity Recognition (Person, Ort, Organisation).
Klassifiziere Meinungen bzgl. Haltung
Es gibt zwei Ansätze, um die Haltung einer Person zu bestimmen:
- Machine Learning: Aus Beispielen lernt ein Algorithmus Wortkombinationen zu verstehen und mit einer Haltung zu assoziieren.
- Wörterbuch: Jedes Wort wird mit einer positiven, negativen oder neutralen Haltung assoziiert, welche gewichtet.
Die meisten Cognitive Services in der Cloud kombinieren beide Ansätze und funktionieren für generische bzw. domänen-unspezifische Anwendungen sehr gut.
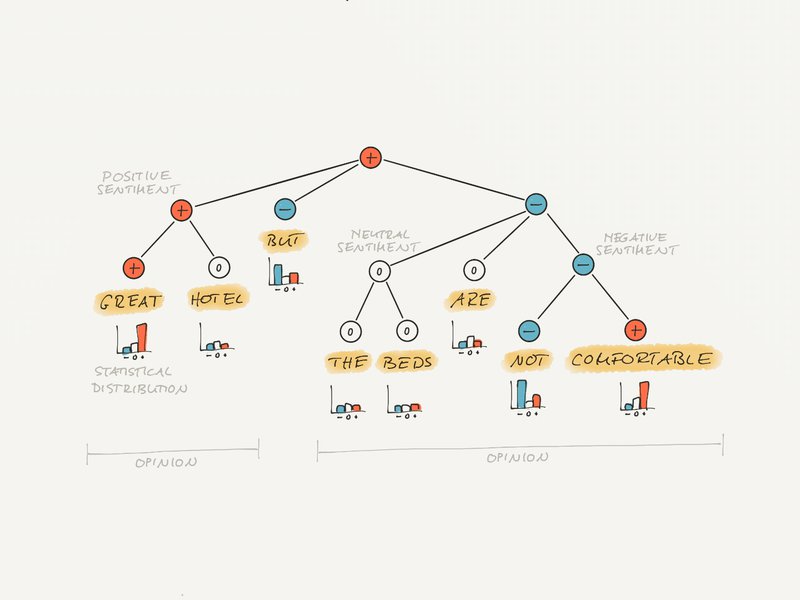
Analysiere und sammle Insights und reagiere darauf!
Aus Kommentaren haben wir Themenfelder identifiziert, Meinungen dazu extrahiert und diese bzgl. Haltung klassifiziert. Darauf basierend können wir nun Insights gewinnen.
Produkt- und Angebotsverbesserung
Anhand der gewonnen Insights können wir definieren, woher die grössten Unzufriedenheiten stammen. Damit wissen wir, was wir bei unserem Produkt bzw. Angebot verbessern sollten.
Zum Beispiel: Kunden sind ständig mit dem City Hotel unzufrieden, was ihre Haltung zur gesamten Pauschalreise negativ beeinflusst. Wir können nun das City Hotel durch ein anderes Hotel in der gleichen Preisklasse ersetzen.
Steigerung Kundenzufriedenheit
Wir können in Real-Time Kommentare bspw. auf Social Media analysieren. Wenn wir einen negativen Kommentar identifiziert haben, können wir ihn einem Themenfeld zuordnen und die Ursache automatisiert eruieren. Daraus können wir Massnahmen ableiten, um die Kundenzufriedenheit wieder zu erhöhen.
Zum Beispiel: Anita schreibt auf Facebook, dass das Bett im Hotel unbequem ist. Wir könnten ihr nun ein zusätzliches Kissen anbieten.
Konkurrenz- und Marktanalyse
Wir können nun das gleiche Verfahren auf die Kundenkommentare für die Konkurrenz anwenden. Daraus können wir wertvolle Insights gewinnen, was Kunden bei der Konkurrenz schätzen und was nicht. Daraus lassen sich Massnahmen ableiten, die uns klarer differenzieren oder das Erfolgsrezept der Konkurrenz kopieren.
Zum Beispiel: Beatrice schwärmt von den Croissants, welche es zum Frühstück in einem anderen Hotel gibt. Wir könnten nun anstelle des normalen Frühstücks-Kaffees einen besonderen Latte Macchiato anbieten.
Zusammenfassung
Ein zufriedener Kunde ist die Basis für Geschäftserfolg. Dazu müssen wir verstehen, was der Kunde erreichen will und was ihn daran hindert. Sein Feedback liefert uns zudem Wettbewerbsvorteile und ermöglicht eine stärkere Positionierung am Markt.
Wir haben dazu drei Geschäftsnutzen beispielhaft umrissen:
- Produkt besser auf Kundenbedürfnisse anpassen, um die Kundenzufriedenheit zu erhöhen.
- In Echtzeit auf Kundenbedürfnisse reagieren. Damit bleibt der Kunde zufrieden, die Chance für Retention erhöht sich und Neukunden können günstiger akquiriert werden.
- Insights bzgl. Alternativen der Konkurrenz gewinnen, um ihren Vorteil zu kopieren oder etwas entgegen zu setzen.
Weitere Beispiele für Geschäftsnutzen sind:
- Customer Churn Prevention, um Kundenabgang zu minimieren
- Smart Assistants, um Kunden-Touchpoints zu verbessern
- Social Listening, um Insights zur aktuellen und allgemeinen Wahrnehmung von Produkten oder Unternehmen zu gewinnen.
Am Besten kann ein Kunde seine Erfahrungen (sein Feedback) in natürlicher Sprache ausdrücken. Solche Aussagen zu analysieren ist aber aufwendig.
Sentiment Analysis ist ein Verfahren, um natürlichsprachliche Aussagen automatisiert zu untersuchen. Themenfelder werden organisiert, Meinungen extrahiert und bzgl. emotionaler Haltung (engl. Sentiment) klassifiziert. Daraus ergeben sich Insights, die erhebliche Wettbewerbsvorteile und Effizienzsteigerungen ermöglichen.
Die Technologien für Sentiment Analysis stehen heute rasch zur Verfügung, insbesondere über die Cloud als Cognitive Computing Services oder Machine Learning-as-a-Service. Mit der fachgerechten Expertise, kann man Sentiment Analysis erfolgreich einsetzen und daraus effektive Geschäftsnutzen ziehen.