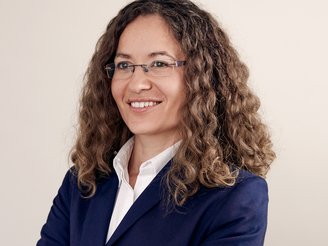
Floarea Serban
IT-Architect
Die Idee hinter Predictive Maintenance klingt vielversprechend: Es werden die Sensordaten einer Anlage dazu genutzt, den Zeitpunkt und die Art der notwendigen Wartungsarbeiten zu bestimmen. Dadurch lassen sich Wartungsprozesse optimieren und kostspielige, ungeplante Ausfälle reduzieren. Der Aufbau einer Predictive Maintenance-Lösung ist aber komplex, mit neuen Technologien verbunden und stellt damit ein nicht zu vernachlässigendes Projektrisiko dar. Eine schrittweise Vorgehensweise, ausgehend vom Minimum Viable Product (MVP), hilft dabei, das Risiko zu minimieren. Dies soll kurz an einem Beispiel erläutert werden.
Autoren: Floarea Serban & Geri Reif
Neue Technologien scheitern oft, weil das Vorgehen, wie sie implementiert werden, nicht optimal aufgegleist ist. Die Komplexität ist enorm und die Unabwägbarkeiten, gekoppelt mit fehlenden Erfahrungswerten, schrecken oft ab. Durch das passende Vorgehen, nämlich eine schrittweise Projektdurchführung, kann ein Grossteil der Risiken deutlich gemindert werden. Denn indem man bei jedem Schritt ein lauffähiges Produkt hat, kann von Fall zu Fall entschieden werden, wie das weitere Vorgehen aussieht.
Zur Veranschaulichung sehen wir uns einen Predictive Maintenance Case in der Bauindustrie an. Dabei werden Baumaschinen mit Sensoren ausgestattet, um Daten wie Ölstand, GPS-Koordinaten, Batteriespannung, Betriebsstunden, etc., an eine IoT-Plattform zu senden. In der IoT-Plattform werden die Daten in der ersten Ausbaustufe zum Speichern an eine Datenbank weitergeleitet und in einem Dashboard übersichtlich dargestellt. In dieser Ausbaustufe hat der Bauhof bereits auf Knopfdruck einen Überblick über den Einsatzort und den Betriebszustand der Baumaschinen.
Im letzten Schritt zu einer Predictive Maintenance-Lösung werden die Sensordaten in der Datenbank mit Hilfe von Mustererkennung und Machine Learning auf Auffälligkeiten untersucht, welche in der Vergangenheit zu Problemen geführt haben. Werden solche Muster erkannt, wird die Stream Analytics Komponente so konfiguriert, dass sie in den einlangenden Sensordaten in Echtzeit diese Probleme erkennt und eine entsprechende Reaktion auslöst. Auf diese Weise kann auf kritische Betriebszustände reagiert werden, bevor ein grösserer Schaden auftritt.
In diesem Blog Beitrag wurde an einem Beispiel gezeigt, wie man Schritt für Schritt eine Predictive Maintenance Lösung umsetzt. Dabei erzeugt jeder weitere Zwischenschritt einen zusätzlichen Business Value. Das MVP wird sukzessive erweitert und die Erfahrung, die mit den neuen IoT Technologien gewonnen wird, reduziert das Projektrisiko deutlich. Die Wartungsarbeiten werden dadurch effizienter und unnötige Kosten fallen weg.