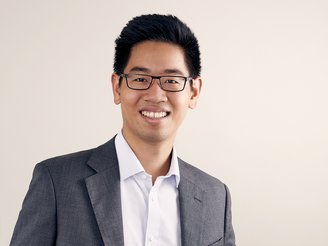
Daniel Yu
Principal Consultant, Director
Die Marketing-Seiten der drei Hyperscaler Amazon, Azure und Google versprechen viel. Was steckt nun wirklich dahinter?
Autor: Andri Lareida
Zusammen mit unserem Kunden entwickeln wir eine Daten-Pipeline zur Klassifikation von E-Mails auf der Basis von Amazon Web Services (AWS). Für die Bearbeitung der Daten setzen wir auf serverlose Lambda-Funktionen, die wir mit Kinesis Data Streams entkoppeln (Grafik 1). Die Machine Learning (ML) Komponente unserer Pipeline wird in SageMaker trainiert und bereitgestellt.
Unser Kunde erhält täglich Tausende E-Mails von seinen Kunden mit Rückmeldungen zum Service oder Anfragen zum Angebot. Das stellt unseren Partner vor zwei grundsätzliche Probleme:
Heute werden die eingehenden E-Mails von Mitarbeitenden bearbeitet und entsprechend kategorisiert. Dies kann bis zu drei Tagen dauern. Um die Kategorien schneller analysieren zu können, entwickeln wir einen ML-Algorithmus, der eingehende E-Mails kategorisiert. In einem zukünftigen Schritt sollen den Mitarbeitenden Vorschläge für die Antwort gemacht werden.
Unser ML-Modell durchläuft seinen Lebenszyklus (Grafik 2) immer wieder:
Dieser Zyklus wird wiederholt, um das Modell an Veränderungen seiner Umwelt anzupassen. Beobachten wir eine Verschlechterung der Performanz, wird der Zyklus neu angestossen und das Modell mit neuen Daten trainiert oder sogar weiterentwickelt.
Amazon SageMaker unterstützt unsere Modelle im Training und in der Bereitstellung. Als vollständig verwalteter Cloud-Dienst bietet SageMaker die Vorteile der Cloud. In unserem Fall:
Keine Fixkosten
Das Training von unserem aktuellen Modell (BERT) verlangt nach leistungsstarken GPUs. Dank der Flexibilität von Cloud-Ressourcen kann auf die Anschaffung teurer Hardware verzichtet werden, die in unserem Fall auch nur für das Training benötigt werden würden.
Skalierung
Unsere Workloads lassen sich gut über mehrere GPUs oder sogar Nodes verteilen. Wir können ein Training beschleunigen, indem wir mehr Cloud-Ressourcen für eine kürzere Periode beziehen. D. h. wir haben zwar doppelte Kosten für die doppelten Ressourcen, brauchen diese aber nur halb so lange. Die Kosten für ein Training bleiben somit fast konstant.
Keine Wartung
SageMaker stellt die gesamte Plattform bereit, somit müssen keine Hardware und Betriebssysteme verwaltet werden. Unser Team kann sich ganz auf die Entwicklung konzentrieren, da so gut wie keine Wartungsaufgaben anstehen.
SageMaker wurde entwickelt, um Machine Learning in der Cloud einfacher zu machen. SageMaker nimmt Datascientists und Engineers repetitive Arbeit ab, damit sich diese auf das Wesentliche konzentrieren können.
In unserem konkreten Projekt benutzen wir SageMaker für das Training unseres Algorithmus und die Bereitstellung des fertig trainierten Modells. Dies nimmt unseren Data-Scientists und -Engineers Arbeit ab, die sie wieder in die Verbesserung des Algorithmus und der Daten-Pipeline stecken können.
Für die Entwicklung stellt uns SageMaker Docker-Images zur Verfügung, die schon mit den nötigen Treibern und ML-Bibliotheken versehen sind. Die Entwickler müssen sich nur noch um das Wichtigste, das eigene Modell, und das Laden der Daten kümmern.
Ist ein Entwicklungsschritt abgeschlossen, wird das Training per Script gestartet. Für das Training müssen das zu verwendende Datenset, Grenzen der Hyperparameter-Optimierung sowie die Ablage der entstehenden Artefakte definiert werden. SageMaker führt das Training mehrfach durch, bis die beste Kombination der Parameter gefunden wird. Das trainierte Modell, sowie die Auswertung der Test-Ergebnisse werden in einem S3 Bucket abgelegt.
Entscheiden wir uns dafür, ein neues Modell in die Produktion zu verschieben, wird das trainierte Modell aus S3 in ein spezielles Serving-Image geladen und an SageMaker übergeben. SageMaker stellt eine Instanz auf EC2 bereit, installiert das Image und konfiguriert einen REST Endpunkt, über welchen das Modell angesteuert werden kann. Wenn gewünscht kann das Modell per Knopfdruck auf mehrere EC2 Instanzen skaliert werden. Der Endpunkt ändert sich dabei nicht und bleibt verfügbar.
Im Wesentlichen sehen wir im Projekt zwei Nachteile:
SageMaker ist das AWS-Framework für Machine Learning und bietet eine Plattform und Werkzeuge zur Unterstützung des gesamten Lebenszyklus eines ML-Projekts. In unserem E-Mail-Klassifizierungsprojekt setzen wir auf SageMaker, um ohne Investitionen und ohne Integrationsaufwand Modelle trainieren und bereitstellen zu können. Dadurch kann sich unser Entwicklungsteam voll und ganz auf die wertschöpfenden Aufgaben konzentrieren. Deshalb sind wir der Ansicht, dass die Vorteile der Cloud und SageMaker die Nachteile klar überwiegen.