QCon London 2020: Drei Trends in Machine Learning & Streaming Data
Die QCon Konferenz in London war eine gute Gelegenheit um zu sehen, in welche Richtung sich die Machine Learning und Data Welt bewegt.
Autor: Valentin Trifonov
Auch dieses Jahr hat die ipt die QCon Konferenz in London besucht. An dieser grossen, industrienahen Software Engineering und Architektur Konferenz waren auch Machine Learning (ML) und Streaming Data vertreten - sogar mit eigenen Tracks. Eine gute Gelegenheit also um zu sehen, in welche Richtung sich die ML und Data Welt bewegt.
ML-as-a-Service
Mit dem wachsenden Cloud-Angebot ist ML nun einfacher nutzbar denn je.
Die grossen Cloudanbieter bieten Dienste für gut erforschte Standardfälle, etwa Recommendation Systems, Spracherkennung, Bilderkennung oder Sentiment Analysis. Diese Dienste sind nur einen API-Aufruf entfernt - damit sind sie nutzbar ohne eigene Modelle, Data Science Kenntnisse und eigener Infrastruktur für Training und Betrieb. Dies nimmt den Entwicklern Arbeit ab. Gerade in Kombination mit Serverless können mit solchen Technologien sehr schnell Prototypen auf die Beine gestellt werden.
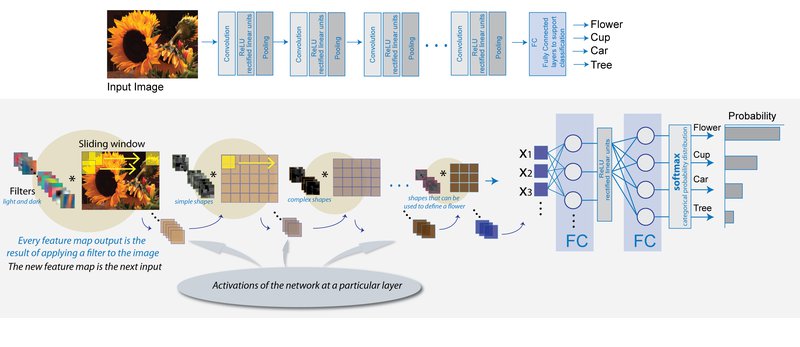
Alle unsere Probleme löst dieser Ansatz jedoch nicht. Die gleichen Eigenschaften können nämlich auch einen Nachteil darstellen: Ein massgeschneidertes Modell, entwickelt auf Basis der eigenen Daten und mithilfe des Domänenwissens, kann eine höhere Genauigkeit erzielen.
Machine Learning at the Edge
Edge Computing sind Berechnungen, die «nahe» am Endbenutzer stattfinden statt zentralisiert in der Cloud. Besonders für IoT-Technologien ist das ein relevanter Begriff. IoT Geräte, die Daten vor Ort bearbeiten, bieten eine höhere Servicequalität. Die Berechnungen sind unabhängig von der Verfügbarkeit des Netzwerkes und von weiteren Diensten im Backend in der Cloud. Zudem können Latenzzeiten stark gekürzt werden. Mittlerweile sind aber auch Datenschutz und Security ins Rampenlicht gerückt und somit starke Argumente für eine Unabhängigkeit von der Cloud.
Machine Learning ist ein besonders guter Kandidat für Edge Computing. Modelle trainieren benötigt viel Rechenleistung. Inferencing hingegen, also Vorhersagen mit einem bestehenden Modell zu treffen, ist sehr viel weniger rechenintensiv und kann in vielen Fällen auf die «Edge» übertragen werden. Es wird prognostiziert, dass sich dieser Ansatz in Zukunft weiter durchsetzt. Der Markt für entsprechende Hardware ist am Wachsen. Das Motto lautet:
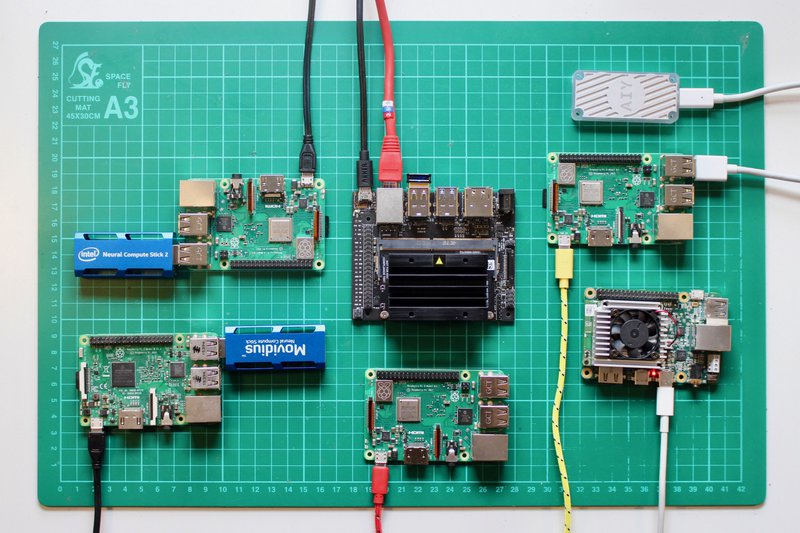
Auch Gartner hat im Hype Cycle letzten Herbst eine ähnliche Vorhersage getroffen. Könnte dies bedeuten, dass in Zukunft die Cloud für den Machine Learning Einsatz an Relevanz verliert?
State of Data Engineering
Machine Learning ist nur so gut wie die Qualität der Daten. Um Daten in Echtzeit aufzubereiten, wendet man sich an Streaming Data Technologien - etwa Kafka, Spark, oder Flink. Diese Plattformen sind hochverfügbar, skalierbar und schnell, jedoch auch sehr komplex. Auch dieses Jahr sehen wir, dass noch viele Data Engineering Herausforderungen offen sind:
- Multi-Tenancy Hell: Mit einer wachsenden Anzahl Jobs auf einem Cluster steigt das Risiko, dass ein fehlerhafter Job den ganzen Cluster abreisst oder Ressourcen für andere blockiert. Vorreiter wie Lyft erzählen uns, wie sie das Problem geschickt lösen, indem sie stattdessen einen eigenen Cluster für jeden Job nutzen. Damit der Betriebsaufwand nicht durch die Decke geht, provisioniert und verwaltet ein Kubernetes Operator die Cluster automatisch.
- Ressourcenverteilung: Welcher Job benötigt wie viel Rechenleistung? Der Ressourcenverbrauch kann stark schwanken, etwa beim Einlesen historischer Daten um einen Job zu starten.
- Die alte und die neue Welt vereinen: Streaming Data löst die Batch-Verarbeitung nicht ab. Vielmehr ergänzen sich beide Architekturen gegenseitig. Nicht nur aus fachlichen Gründen werden Daten oftmals archiviert oder batch-basiert verarbeitet. Im Fehlerfall müssen Berechnungen jederzeit in Zukunft wiederholbar sein. Beide Architekturen müssen also koexistieren - auch bekannt als Lambda-Architektur.
Interessante Entwicklungen in dem Bereich sind etwa Autoscaling (zum Beispiel Google Cloud Dataflow), oder eine gemeinsame Abstraktion für Batch und Streaming (zum Beispiel Apache Beam).
Zukunft
Wohin bewegen sich Machine Learning und Streaming Data nun, in die Cloud oder von der Cloud weg? Dies hängt stark vom Use-Case ab. Sicherlich ist es möglich, dass wir in Zukunft weiterhin Trends in beide Richtungen beobachten. Wir sind gespannt wie sich diese Themen mit ausreifendem Tooling entwickeln.