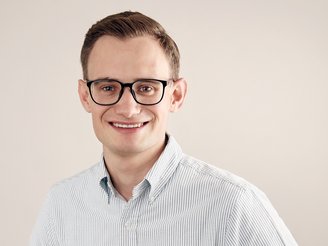
Nicola Storz
Principal Consultant, Director
Blogserie | From Data to Business Value with Data Mesh | #2
Autoren: Nicola Storz & Yves Brise
“Für einmal muss man kein neues Produkt lizensieren” war die gute Nachricht in unserem ersten Blog in der Serie zu Data Mesh. Das hören IT Manager gerne. Der Nutzen, den es verspricht, ist ebenfalls ansprechend: Mehr Data-Drive in die Organisation bringen und den ROI der Investitionen in die IT Infrastruktur realisieren. Doch was ist jetzt das Data Mesh, wenn es kein Produkt ist?
Data Mesh ist ein Organisationskonzept für Daten und für die Organisation, die die Daten bewirtschaftet. Es stammt von Thoughtworks [Data Mesh, ISBN: 978-1492092391] und ähnelt vom Prinzip her dem Ansatz des Domain Driven Design, der in der Softwareentwicklung schon länger in Gebrauch ist. Der Grundgedanke: Dezentralisierung der Daten, maximale technologische Unterstützung durch eine Plattform und minimale zentrale Governance, um die Interoperabilität zu gewährleisten und einen Scale-Out für Daten zu erreichen.
Data Mesh ist zur Zeit einer der am meisten diskutierten Hype-Begriffe in der IT und insbesondere in den Daten- und Analytics Kontexten. Das ist für uns Grund genug, etwas Licht ins Dunkel zu bringen. Ein Data Mesh basiert unabhängig von der Technologie auf vier Prinzipien, die wir hier erklären und anhand eines Beispiels illustrieren (https://martinfowler.com/articles/data-mesh-principles.html).
Die Verantwortung für die Daten soll von der Domäne übernommen werden und liegt nicht länger bei einem zentralen DWH oder Data Engineering Team. Die operativen Domänenteams übernehmen selbst die Verantwortung für ihre Daten und stellen diese bereit. In einem Unternehmen kann so das Vertriebsteam seine Verkaufsdaten über seine Domäne hinaus weiteren Teams bereitstellen.
Dabei werden die Daten für zwei Zwecke bereitgestellt: Zum einen für die operative Verwendung in domänen-fremden, operativen Systemen und zum anderen für analytische Zwecke.
Um für Daten das Product Thinking anzuwenden muss das Datenprodukt einer Domäne gewisse Qualitätsmerkmale erfüllen. Daten werden nicht länger als Rohmaterial behandelt, das per ETL bezogen, bereinigt und dann strukturiert bereitgestellt wird. Das Datenprodukt muss vertrauenswürdig, gut strukturiert, über Domänengrenzen hinweg verständlich und auffindbar sein.
Für unser Vertriebsteam heisst dies, dass sie die Verkaufsdaten nach Verkaufsvertrag gruppieren, die Vertrags-ID als Schlüssel setzen und die Angaben zum Vertrag verständlich strukturieren. Sie garantieren die Qualität und Aktualität der Daten per SLA. So können sie beispielsweise in einem analytischen Use-Case mit den Kundendaten assoziiert werden.
Damit der Datenaustausch zwischen einer Vielzahl von Teams skaliert, müssen die Infrastruktur zur Bereitstellung der Daten sowie die Datenprodukte selbst bereitstehen. Self-Services sind dabei zentral für die Infrastruktur und die Datenprodukte selbst.
Bei der Bereitstellung der Vertriebsdaten kann das Vertriebsteam in unserem Beispiel direkt per Self-Service die Plattforminfrastruktur bestellen, damit ihr Datenprodukt entwickeln und das Produkt zur Konsumation anbieten. Das Team Kunden-Analytics findet die Vertriebsdaten im Datenkatalog und kann sie per Self-Service Anfrage bestellen. Die Daten können sie mit den Kundendaten korrelieren, um aus diesen Daten das Verkaufspotenzial pro Kunde zu errechnen und diesen Wert ihrerseits wieder als Datenprodukt anbieten. Dabei ist wichtig, den Benutzern die richtigen Werkzeuge in die Hand zu geben, die auf deren Bedürfnisse und Skill Sets passen.
Damit die ersten drei Prinzipien nahtlos ineinandergreifen, ist die Einhaltung gewisser Standards erforderlich. Diese werden von einem domänenübergreifenden Gremium besprochen und durchgesetzt. Dazu gehören Anforderungen an die Struktur und Schnittstellen der Datenprodukte, Namenskonventionen, die Versionierung, die Dokumentation und die Datensensitivität. Der Zugriff auf die Daten wird über Policies abgesichert.
Für unser Vertriebsteam bedeutet dies, dass die Bereitstellung des Datenproduktes über eine Standardbeschreibung (z.B. Async-API Spezifikation) zu erfolgen hat. In dieser Spezifikation muss der fachliche Data Owner, die Domäne “Vertrieb” und die Namensgebung angegeben werden. Die Kundenreferenz “Kunden-ID” erhält dann von der Kundendomäne denselben Namen wie in der Vertriebsdomäne. So kann das Team Kunden-Analytics diese Daten später korrelieren, um das Verkaufspotenzial zu errechnen.
Die vier Prinzipien ermöglichen es Domänenteams, die Verantwortung für ihre Daten zu übernehmen und zwischen verschiedenen Domänen auszutauschen. Der Nutzen der vorhandenen Daten kann gesteigert werden, indem Datenprodukte in guter Qualität und korrelierbar zur Verfügung stehen.
In der Softwareentwicklung ist der Begriff DevOps bekannt geworden. Software Teams sind nicht nur für die Entwicklung, sondern auch den Betrieb und den Support ihrer Software zuständig. Bei Data Mesh gibt es im Prinzip das gleiche Vorgehen mit Daten (https://learning.oreilly.com/library/view/data-mesh/9781492092384/). Dieses Prinzip auf Daten anzuwenden, wurde unter dem Namen DataOps bekannt. Data Mesh ist in gewisser Weise eine Weiterentwicklung davon und ermöglicht eine Skalierung von DataOps.
Die Organisation muss zwei Sachen zur Verfügung stellen, damit Data Mesh erfolgreich implementiert werden kann:
Ein zentrales Plattformteam, welches die Werkzeuge für die DataOps Teams zur Verfügung stellt und möglichst viel davon automatisiert (Prinzip 3). Ausserdem ist dieses Team ein guter Ort, um die föderierte Governance zu verankern (Prinzip 4).
Fähige Domänenteams mit den richtigen Skills, die ihre eigenen Datenprodukte definieren und bereitstellen können (Prinzip 1 und Prinzip 2).
Wir haben in diesem zweiten Beitrag der Blogserie erklärt, was ein Data Mesh ist. Die vier Prinzipien von Data Mesh sind eingängig und einfach. Wie man das Nutzenversprechen aus dem ersten Blog nun tatsächlich realisiert und technologisch umsetzen kann, werden wir demnächst in Blog 3 beleuchten. Ausserdem gehen wir in Blog 4 darauf ein, wie wichtig es ist die Menschen in der Organisation einzubeziehen und wie die Teams dafür organisiert sein sollten.
Blog #1 die Motivation dahinter
Blog #2 ihre vier Prinzipien
Blog #3 die technische Umsetzung
Blog #4 ihre Teams
Data Mesh, ISBN: 978-1492092391
https://learning.oreilly.com/library/view/data-mesh/9781492092384/
Blog Thoughtworks
Wir freuen uns auf Deine Kontaktaufnahme